Fragrance and flavor substances are strong-smelling organic compounds with a pleasant odor (fragrance chemical) or a pleasant taste (flavor chemical). A fragrance substance is used as a component in a perfume or a cosmetic product, while a flavor substance is used to enhance the flavor of beverages and food products. The threshold of olfaction presents a key feature to all the odor active compounds and can be assessed by virtual screening methods using machine learning (ML) models. Such techniques establish correlations between the chemical information obtained directly from the structure of the molecule and the physical, chemical, biological or environmental properties of the compounds. These ML generated models aim to predict physico-chemical properties of non-synthesized compounds based on the knowledge of their molecular structure represented by so-called descriptors, e.g., molecular weight, number of functional groups or atom types, electronegativity and many more. ML modeling has proven extremely useful in a broad variety of applications for predicting catalytic activity, rheological properties, solubility, corrosion inhibition, the influence of additives, surfactant properties, etc.
The Challenge
An odor is an impression in the brain obtained by the detection of a volatile component at a very low concentration by odorant receptors that is perceived by the sense of olfaction of humans or animals. However, two chemicals having the same odor threshold may not produce the same level of annoyance in the surroundings. This demonstrates the existence of a complex mechanism of action of the odorant receptors. Moreover, an odorous molecule present in the environment can bind to several odor receptors at a time. This ambiguous nature of odorant receptors urged research efforts in developing machine learning models that enable the prediction of the odor threshold of compounds and provide insights into their binding possibilities without costly and time-consuming experimental setup. Prerequisite, however, is the availability of a sufficiently large consistent data set that can be used to train and validate the machine learning model.
The Work
In the present case study, machine learning modeling was carried out for establishing a relationship between the odor threshold data set of 53 compounds comprising different aliphatic alcohols, for which the odor detection threshold is known, and their structural properties. Different types of descriptors belonging to the constitutional indices, functional group counts, the extended topochemical atom (ETA) indices were calculated. The first two types of descriptors include topological, structural, physicochemical, electronic and spatial types, whereas the ETA descriptors provide information about the electronic features, size, shape, branching, and functionality of molecules, along with the electron richness, unsaturation, polar surface area and ability of hydrogen-bond formation. The calculations were performed using the alvaDesc plugin within the MAPS platform.
The Results
The data set was divided into two classes: one comprises 42 compounds considered as the training set and the other 11 compounds were assigned to the test set. The training set was used for model development and the test set for subsequent model validation. This division is very important as it determines the quality of the QSPR model. The final QSPR models were selected based on the significant values of different statistical parameters: determination coefficient (R2) close to 1, small RMSE and a small number of descriptors to avoid overfitting of the model, which would restrict its predictability.
Figure 1 shows the experimentally observed vs. calculated/predicted responses of log (1/T) for all the compounds in the training and test sets. The ETA indices are found to be the most important descriptors for predicting the odor threshold as they possess sufficient diagnostic power in defining the changes in the property values with variation in the structure of compounds with –OH functional groups. They are simple, easy to interpret and require less time for calculation. The positive sign in the coefficient of the descriptor indicates that the log (1/T) increases with the increase in the molecular size of alcohol. This is correctly observed for the highest potent molecule in the set C16 (1-decanol). The molecular branching and electronic parameters significantly influence the odor potency. Moreover, increased lipophilicity and reduced electronegativity increase the odorant property.
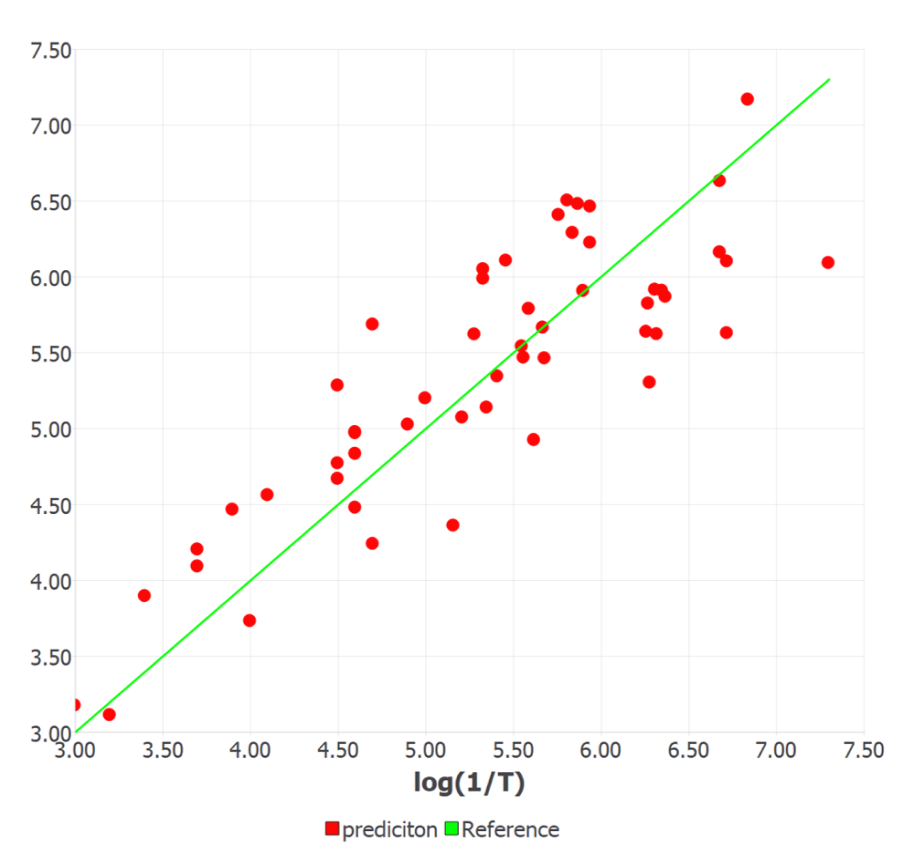
Figure1. Experimental vs. machine learning predicted responses of log (1/T) for 53 aliphatic alcohols.
QSPR modeling can efficiently support and guide experimental work by creating virtual variants of target compounds, predict their properties, and identify the most promising candidates, which saves cost and development time.
- Pal, P.; Mitra, I.; Roy, K. QSPR Modeling of Odor Threshold of Aliphatic Alcohols Using Extended Topochemical Atom (ETA) Indices, Croat. Chem. Acta 2014, 87, 29–37.