Industrial applications of materials science require simulations of large systems which imply the use of accurate force-fields. Despite the availability of a wide range of force-fields the complexity of the systems to be treated, or the conditions considered, highlight the limitations of these force-fields. This situation is a barrier to a wider use of materials simulations by industrial users. On the other hand, developing specific force-fields requires strong knowledge of optimization techniques and is cumbersome. Recently various implementations of machine learning techniques have been developed. However, these techniques produce interaction data that lack physico-chemical content. In Scienomics we have adopted in MAPS 5.0 the recent machine-learning method developed by Prof. Edward Maginn from Notre Dame University. In this webinar we will present the methodology and showcase the refinement of Amber Cornell force-field in order to predict accurately the interfacial tension and the temperature dependence of thermal conductivity and density of industrial relevant systems such as surfactants and hydrocarbons.
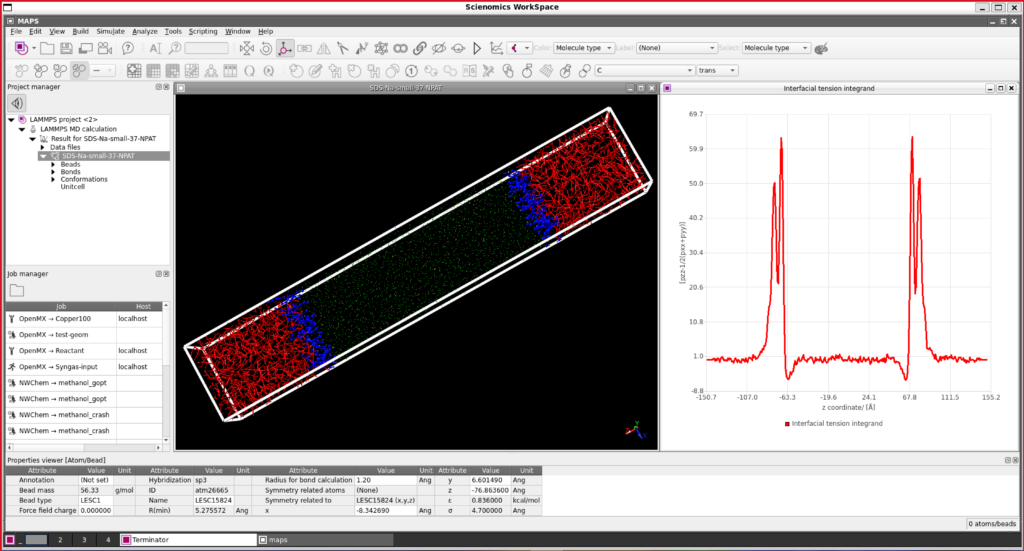
MAPS 5.0 interfacial tension calculation in mesoscopic representation of SDS in Water and Oil. In blue, SDS surfactant, in red Hexadecane (Oil) and Water is located in the middle section of the model.
A Machine Learning-Directed Optimization Method for Developing Accurate and Transferable Force Fields
Edward Maginn
Keough-Hesburgh Professor
Department of Chemical and Biomolecular Engineering
University of Notre Dame
Notre Dame, IN 46556 USA
Abstract: Accurate force fields are necessary for predictive molecular simulations. However, developing force fields that accurately reproduce experimental properties is challenging and time consuming. In this talk, I will describe a machine learning directed, multi-objective optimization workflow for force field parametrization that evaluates millions of prospective force field parameter sets while requiring only a small fraction of them to be tested with molecular simulations. The approach is general and enabled us to identify multiple low-error parameter sets for two distinct test cases: hydrofluorocarbon vapor−liquid equilibrium and an ammonium perchlorate crystal phase. I will show that these force fields, because they capture the physical interactions present in the system, can accurately model a wide range of thermophysical properties not used in the training procedure, such as viscosity, thermal conductivity, and Joule-Thomson coefficients. Finally, I will describe how we are extending the workflow to develop generalized force fields for mixtures and for computing properties of new molecules where no experimental data exist.
This work was supported by the US National Science Foundation, Award numbers OAC 1835630, CBET 1017474, EFRI DChem 2029354, and CBET 1917474 as well as the Air Force Office of Scientific Research under Contract AFOSR FA9550-18-1-0321.